01 Baseline
Coverage and Completeness
Attribution and Accuracy
Change and Latency
02 Assessment
Adoption
Performance
Baseline Assessment are non invasive - provide your datafeed and mapping to alignVu and we do the rest.
Baseline Assessment are a one time, fixed priced service that runs for one month and delivers a rapid assessment of your datafeeds alignment and lineage between your data platforms – both multiple external and internal datafeeds – that tracks data attribution through lineage and identifies gaps, discrepancies and transformation problems. Baseline Assessment also provide impact assessment of change, migration (data and platform) and can also run comparative assessments between datafeeds where you’re seeking to rationalise or single source.
End-to-End Alignment
If you want an end-to-end alignment as one which can provide seamless migration and upgrade capabilities, we add your datafeeds mapping from the alignVu Translation data model to your platforms schema. In this we build Alignment(s), profiling the data at field, attribute and value level to verify and attest that it can meet the target platform requirements and connect you to any and all of our pre-existing Alignments (subject to license)
Process
- Verify the content at field and attribute level across our aligned source – tracking change and latency between the chosen datafeeds
- Compare the number and values of each field in our translation model alignments with your mapping using the fields in the common datafeed you have mapped from and provide a detailed analysis
- By entity type (both datafeed and Model entity types)
- By attribute, field, datafeed, datafeed group and datafeed product
- By timelines – handing changes, latency difference
Deliverables
- Detailed comparative analysis across each datafeed Aligned for comparable coverage, accuracy and completeness – by value, by numbers and specific field by field value comparisons GAP analysis for mapping differences (if any) which create, as a result, a difference or omissions in the data being populated by the client mapping compared with the translation and the data supplied by the Aligned datafeed
- Detailed report
- On screen dashboards and assessment and ability to switch on as a service
Example Case Study: A Baseline Assessment of Dow Jones Risk Lists for Payments and Transactions Screening Product
01 Baseline - Alignvu’s approach
Alignment in:
Each datafeed had been mapped into a common data model and Alignments created – the cross alignments are compared
Translation Data Model:
Each datafeed was then translated to the alignVu data model, and each value within each field was compared to one another to confirm whether each datafeed had the same number of values in each field? Are they the same exact values? And can each specific value (i.e. each name) be attributed to its original creator?
alignVu used this data to create a comparison between LPTS at attribute level and record level; we used the cross-references to identify both overlap and where there was a discrepancy.
Change and latency are slightly differing concepts in that the assessment defines a change as when an update was first made, i.e. published vs latency in that update being made available to the end-user via the electronic datafeed and whether that latency is within the update SLA for a specific data feed.
Baseline: Coverage and Completeness
Baseline: Attribution and Accuracy
Baseline: Change and Latency
Coverage and Completeness
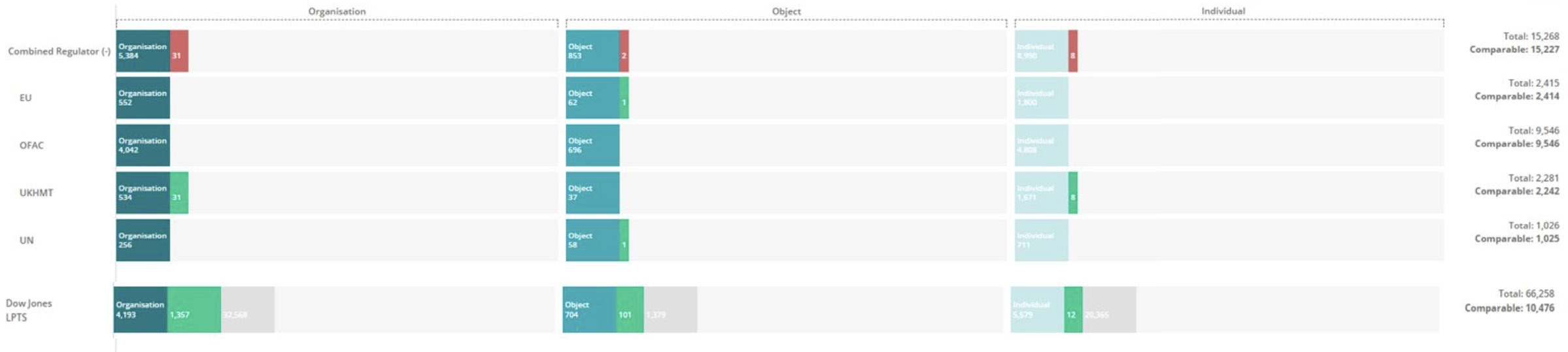
Throughout our evaluation, from the point in time when a regulator published or sent notification of a sanction update, LPTS was consistently updated in advance of the regulators own datafeeds.
Any gaps between LPTS and a regulator notification we identified were applied to LPTS within the product SLA and therefore classified as latency. There were in no other coverage gaps identified between the LPTS datafeed and the regulator notification.
Where sanctioned entities found in LPTS were compared against the time they were published by the regulators on their websites or notified by email, there is a time delay between appearing on the regulator notification and being made available on LPTS. Surprisingly, there was an even longer and variable delay between regulator notifications being made available by the regulator on their own datafeed. Sometimes the delay was beyond the evaluation period of the study. We found that LPTS added regulator data in advance of the regulator datafeeds in this benchmark as a consistent outcome through the 30-day evaluation period of the baseline
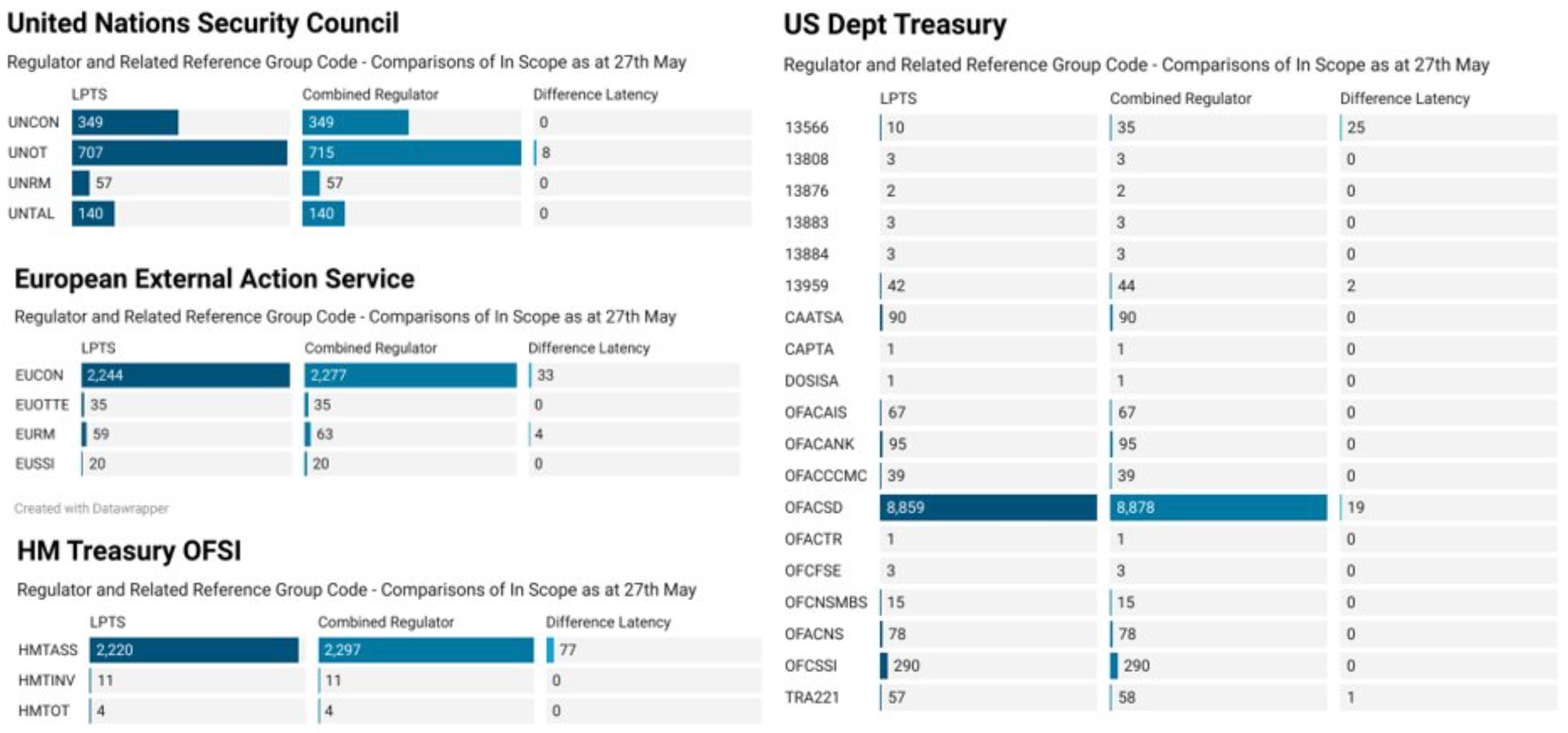
Each reference code group in the combined regulator datafeed reconciled to its LPTS equivalent; in fact, we found more valid lists in LPTS then we did in the Regulator files.
The reason for the differences are a combination of latency or omission, omission being where there is a regulator enforcement
published that has been applied by the LPTS editorial team, but not added to the automated regulator feed at the point of the
baseline from 27th May through to 30th June.
Attribution and Accuracy
Results attribution of field values and comparability of values
Combined with completeness of records, the assessment established attribution at each value in each field level back to the creator of the content defined by adding a reference group code (multiple where a value is from more than one regulator). In fact, LPTS was so accurate that we found examples where LPTS held a name twice (with separate attribution) due to punctuation differences between regulators (comma v period).
We found no anomalies or gaps, which provided a high degree of transparency and confidence in the data.
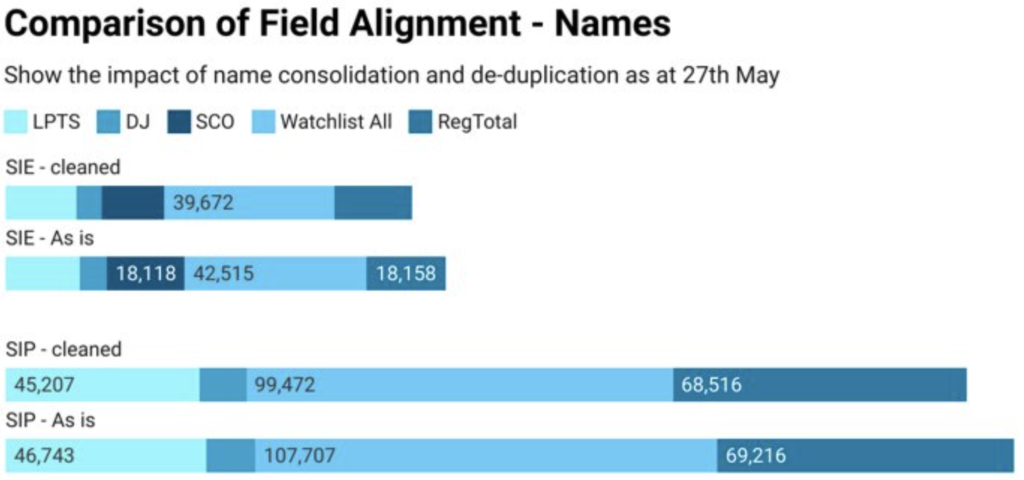
Change and Latency
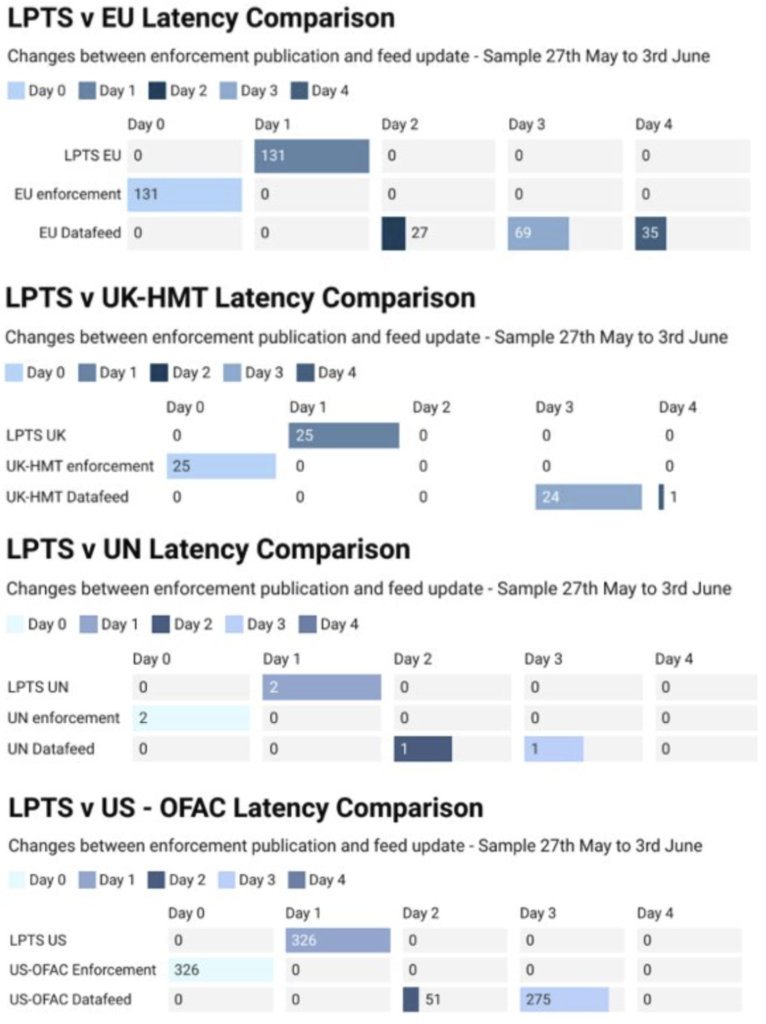
Day 0 is based on the release date by the regulator through publishing a notice on the regulator website and/or receipt of email notification.
We subscribe to each regulator (but only consume data when provided by the regulator automated feed), and the latency comparisons above reflect Day 0 = enforcement notification. We then run a daily comparison between LPTS and each regulator to compare electronic data feeds and it is only when provided in the automated data feeds does this report count the latency difference. So Day 1 is any overnight update as we compare LPTS and each Regulator as at 6am daily, day 2 is the next daily update from the 11.30pm LPTS file compared with the regulators feeds downloaded at the same point in time.
In the sample data set over the period of the benchmark, LPTS data feeds were between 1 and 2 days in advance of the automated regulator data feeds in providing updates covering adding new entities, changes to existing entities and delisting of entities.
As can be seen from the sample results, the regulator feed updates are between 1 and 2 days after LPTS is updated, even though changes are communicated by various methods by each regulator prior to the LPTS update.
Each update relates back to the reference group from which the enforcement update was applied to enable Dow to provide its clients with complete, timely data feeds that can also provide detailed audit information as to who updated what and when they updated.
01 Baseline - Alignvu’s approach
Alignment in:
Each datafeed had been mapped into a common data model and Alignments created – the cross alignments are compared
Translation Data Model:
Each datafeed was then translated to the alignVu data model, and each value within each field was compared to one another to confirm whether each datafeed had the same number of values in each field? Are they the same exact values? And can each specific value (i.e. each name) be attributed to its original creator?
alignVu used this data to create a comparison between LPTS at attribute level and record level; we used the cross-references to identify both overlap and where there was a discrepancy.
Change and latency are slightly differing concepts in that the assessment defines a change as when an update was first made, i.e. published vs latency in that update being made available to the end-user via the electronic datafeed and whether that latency is within the update SLA for a specific data feed.
Assessment: Adoption
As a premium product, what does LPTS offer Dow Jones Risk and Compliance customers that they couldn’t obtain directly from the regulators own electronic data feeds.
Assessment: Performance
The ‘so what’ tests insofar as a what impact, if any, can LPTS have in enabling users of the LPTS Product to be able to screen their payments and transactions in a fast and more accurate manner.
Adoption
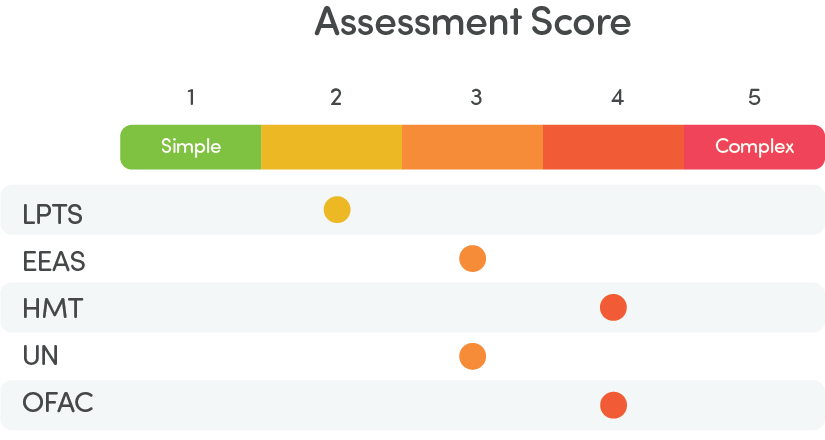
Alignment of LPTS to the AlignVu Translation datamodel was a straightforward single datafeed mapping process.
In comparison, each regulator datafeed had multiple format versions; each Regulator in this study applied different structures and within their own various formats included different levels of data in differing timelines. LPTS, through mapping so many regulators to its consolidation model, makes decisions to combine multiple fields found in a regulator feed to a single LPTS field. LPTS loses points as the relationship between these is not defined (other then within the database) and in general, documentation is subject to interpretation.
LPTS benefits from a strong editorial and curation process, which inevitably involves a significant manual intervention to consolidate each diversity of approach from each regulator (within formats) to the Dow Jones Data model. This simplifies the adoption of sanction feeds, more so the number of regulators a firm needs to validate against. LPTS curation from publication results in the regulators
often having latency issues when compared with LPTS, which combined with attribution back to source provides clear benefits to consumers reliant on clean, accurate sanction and related data.
LPTS consolidation of multiple regulator datafeeds records into a single record results in significant de-duplication of data, with combined with clear reference code based attribution of each name, address, identifier means consumers can refine the specifics they require for their screening use cases in a transparent and auditable manner for example within our study we established that compared with the Combined Regulators in this baseline, LPTS, reduced SIE (organisation and object) names terms reduced by approximately 6% (this increased to 9% when punctuation differences were removed) and SIP (individuals) through de-duplication of names terms reduced by 34%.
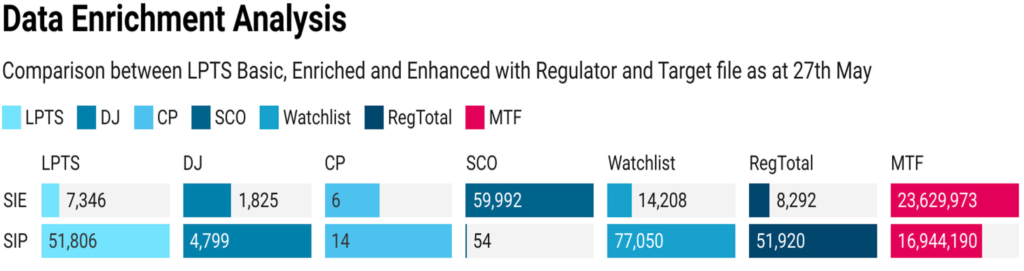
As can be seen here, additional attribute data that ‘fill in the gaps’ have a ‘DJ’ reference group code and can be included or excluded dependent on the screening use case.
The Sanction Control and Ownership (SCO) is where the LPTS datafeed has been enhanced with Dow Jones R&C proprietary data set for entities owned or controlled by sanctioned subjects.
The next section of Assessment: performance used 3 versions of LPTS to establish the value to the end consumer of these optional additions.
Data Enrichment
There is a direct correlation between the completeness of data attributes on each entity being screened and those entities they are being screened against. Within the OFAC screening guidance where there is sufficient relevancy in terms of name, the guidance states:
‘Compare the complete sanctions list entry with all of the information you have on the matching name in your transaction. An entry often will have, for example, a full name, address, nationality, passport, tax ID or cedula number, place of birth, date of birth, former names and aliases’ – if the record is missing a lot of this information for the name in the transaction – then the client should create a SAR and get more information to make a decision and then compare your complete information against the entry. If the information is there, then
• If the information is the same, then it’s a positive SAR
• If different, then it’s not a valid hit, the SAR is classified as a ‘false positive’, closed and no further action is required.
We have confirmed accurate field attribute alignment in both coverage and quality for LPTS Core v the in scope regulators. We found consolidation of fields classed as qualifiers (identifiers and weak alias used to aid decision on a hit) in line with de-duplication as expected.
Additionally, we noted significantly more enrichment in Watchlist (a separate product to LPTS) that covered a number of attributes that we would expect to existing in customer files, such as industry sector codes and which were included in the MTF and used as qualifiers in the screening rules
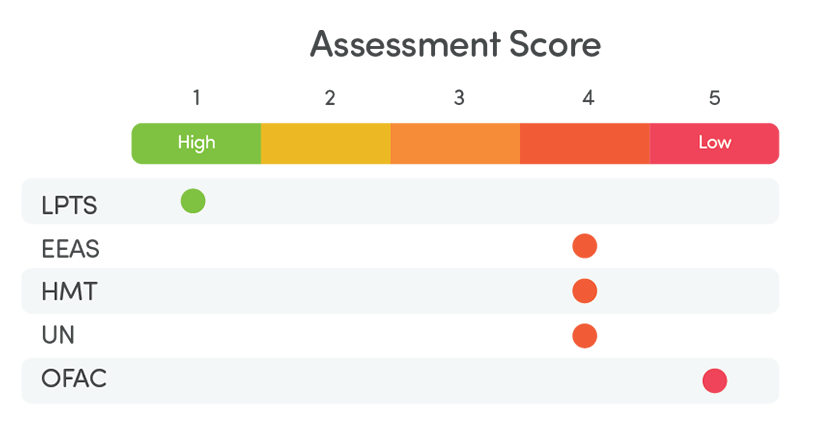
Alignment of LPTS to the AlignVu Translation datamodel was a straightforward single datafeed mapping process.
In comparison, each regulator datafeed had multiple format versions; each Regulator in this study applied different structures and within their own various formats included different levels of data in differing timelines. LPTS, through mapping so many regulators to its consolidation model, makes decisions to combine multiple fields found in a regulator feed to a single LPTS field. LPTS loses points as the relationship between these is not defined (other then within the database) and in general, documentation is subject to interpretation.
LPTS benefits from a strong editorial and curation process, which inevitably involves a significant manual intervention to consolidate each diversity of approach from each regulator (within formats) to the Dow Jones Data model. This simplifies the adoption of sanction feeds, more so the number of regulators a firm needs to validate against. LPTS curation from publication results in the regulators
often having latency issues when compared with LPTS, which combined with attribution back to source provides clear benefits to consumers reliant on clean, accurate sanction and related data.
LPTS consolidation of multiple regulator datafeeds records into a single record results in significant de-duplication of data, with combined with clear reference code based attribution of each name, address, identifier means consumers can refine the specifics they require for their screening use cases in a transparent and auditable manner for example within our study we established that compared with the Combined Regulators in this baseline, LPTS, reduced SIE (organisation and object) names terms reduced by approximately 6% (this increased to 9% when punctuation differences were removed) and SIP (individuals) through de-duplication of names terms reduced by 34%.
Performance
Time to run comparable screening rules to screen each regulator and LPTS against a sample manufactured transaction file (MTF) of 5.5m transactions.
LPTS Core Service ran significantly faster in repeated tests when compared to the same data set in the combined regulator file – averaging around 40% faster processing using the same field values.
The de-duplication of common entities, when compared to the regulator data sets, had a significant impact on the performance of this data set.
Even with LPTS enhanced with DJ reference group data) and LPTS 3 enriched with DJ reference group and Sanctions Control & Ownership (SCO) – in this benchmark, we ran in scope reference groups for like-for-like comparisons) we observed comparatively faster screening performance where enrichment had been applied by Dow Jones.
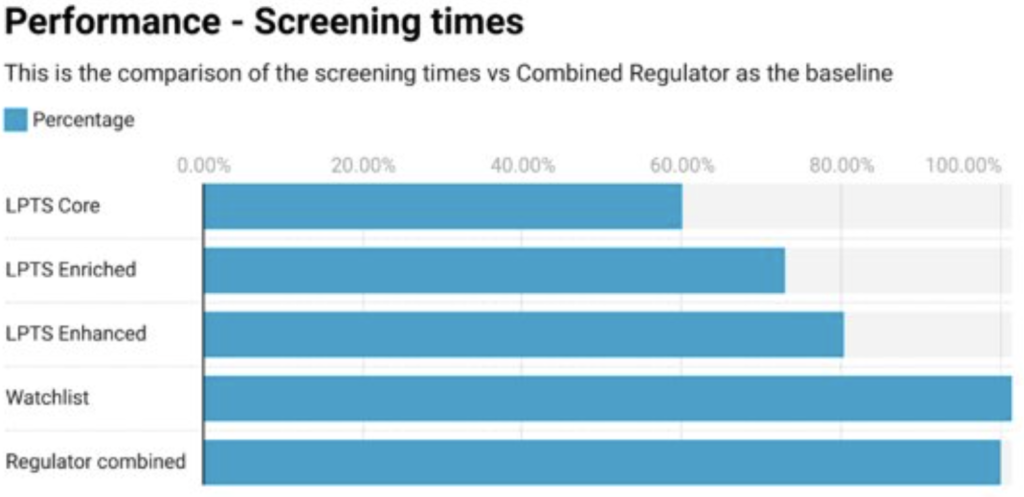
Screening standard OFAC rules against an MTF constructed to ensure an accurate baseline through being able to assess screening performance benchmark against a minimum expected set of results. The MTF contains two copies of each regulator record, one in its exact form as it exists in the combined regulator file and a second version that has had the address and identifier data changed. As a minimum, each LPTS and Regulator file must generate both a:
‘Positive’ hit – each exact copy of the regulator records should be an exact match.
‘False positive’ hit – the second copy of the regulator records should generate a positive hit (name) and then have a ‘false positive’ applied to auto-close the Suspicious Activity Report (SAR) created by the ‘positive hit’.
LPTS delivered a significant efficiency gain in real terms – on a like-for-like basis, it reduces the number of raw SARs by 28% and the number that have to be reviewed by 37%. This improved further with LPTS Enrichment and Enhancement.
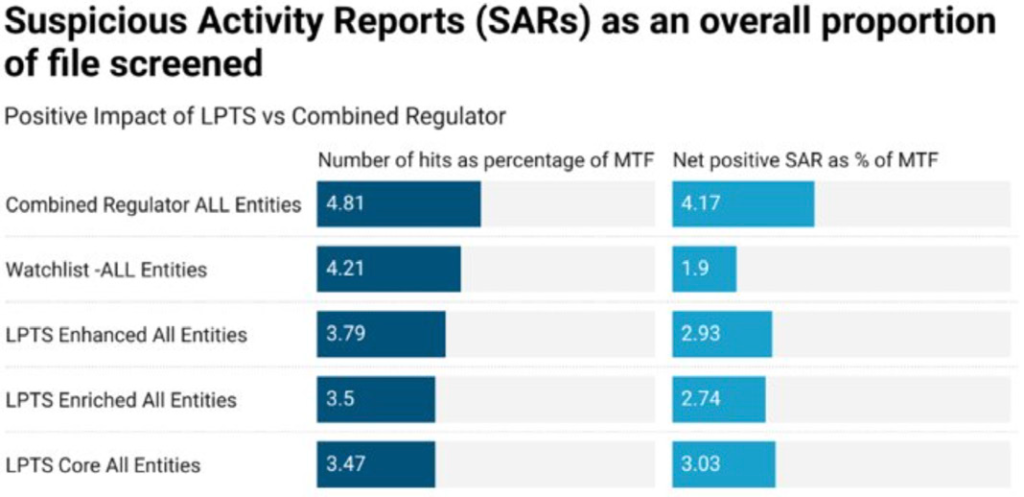